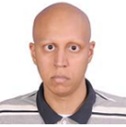
Hamad Alduaij
Kuwait institute for scientific research, Kuwait
Title: Analysis of RC beam with a hole using artificial neural network
Biography
Biography: Hamad Alduaij
Abstract
Reinforced Concrete (RC) is the most widely used material in beams and columns of buildings, bridges and other infrastructures. Behavior of RC beam is a well examined and explained by several laboratory experiments. RC material is highly nonlinear and mathematically complex to model. Finite element analysis is employed by engineers and researchers to predict the nonlinear behavior of RC columns and beams along with laboratory experiments. However, the method, in general, computationally expensive for RC beams and columns due to convergence and other issues. Artificial intelligence techniques such as neural networks can be used to obtain a relatively simple mathematical model describing the behavior of RC beam.
RC beam with a vertical hole is not a well examined subject and it is even more complex to predict using finite element model. To carry out laboratory experiments with various position of the hole along the length and width of the beam and size of the hole is a laborious and expensive task. In the present study, the characteristics of a RC beam with a vertical hole is examined through the use of Artificial Neural Networks (ANN). In this study, limited number of laboratory experimental and finite element results are used to predict the behavior of RC beam with a vertical hole using ANN. Effect of hole with various position along the length and width of the beam and due to change of size of the hole. The analysis was created with a hidden layer of 15 neurons using Bayesian regularization backpropagation algorithm. Bayesian regularization minimizes a linear combination of squared errors and weights. It also modifies the linear combination so that at the end of training the resulting network has good generalization qualities. The network achieved almost 1 r-squared value for both training and testing set. Figure 1 depicts neural network model of the maximum force as the hole moves through the x axis.From the network simulation It was observed that, beam hole near the mid span reduces the load carrying capacity by 20 %. The optimal location of the vertical hole in between the end support and mid span. There is drastic reduction in load carrying capacity is observed if the edge distance between circumference of the hole and beam edge is less than 30 mm.